07.11.2024
12:05 - 12:50
Uhr
Vortrag
Test & AI
Congress Center A
Taras Holoyad
Bundesnetzagentur
Prüfung von KI-Fähigkeiten im Lichte des KI-Gesetzes
Wie auch bei herkömmlicher Software hängen Prüfkriterien für Systeme mit implementierten Algorithmen der künstlichen Intelligenz (KI) bedeutsam von zu testenden Funktionen ab. Sobald ein System unter die europäische Regulierung zu künstlicher Intelligenz fällt, wird eine konkrete Prüfung der Funktionen von KI-Systemen unabdingbar. Ausgehend von aktuellen Normungsarbeiten zu KI wird in Bezug auf Funktionen, die auf Grundlage von Algorithmen umgesetzt werden können, von Fähigkeiten (engl. „Capabilities“) gesprochen. Aufgrund des derzeitigen Mangels an eindeutiger Definition für den Begriff „Fähigkeiten“ bzw. „Capabilites“ im KI-Kontext, zielt der hiesige Konferenzbeitrag darauf ab, Klarheit in Beschreibung und Prüfung von KI-Fähigkeiten zu schaffen. Nach aktuellem Stand wurden in Zusammenarbeit mit Wissenschaft und Industrie die KI-spezifischen Fähigkeiten in einem breiten Spektrum innerhalb der Übergruppen „Wahrnehmen“, „Verarbeiten“, „Handeln“ und „Kommunizieren“ identifiziert [1]. Da Fähigkeiten die Funktion eines Systems widerspiegeln, ist die Formulierung von Prüfkriterien von der zu testenden Fähigkeit abhängig. Beispielsweise kann in diesem Zusammenhang im maschinellen Sehen die Prüfung der Korrektheit von der Fähigkeit „Klassifizierung“ von Objekten auf Bildern mit der Metrik „confidence score“ erfolgen.
Vor diesem Hintergrund ist im Konferenzbeitrag eine Testbeschreibung für Systeme mit implementierten Methoden des maschinellen Lernens (ML) vorgeschlagen, unter Beachtung der Anforderungen aus dem europäischen KI-Gesetz. Grundsätzlich ist die vorgeschlagene Testbeschreibung zur Prüfung der Fähigkeiten von ML-Systemen konzipiert, in Bezug auf die Gütekriterien „Correctness“, „Robustness“, „Avoidance of unwanted bias“ und „Security from adversarial attacks“. Um die praktische Anwendbarkeit zu demonstrieren, wurde die Testbeschreibung auf das Computer-Vision-Modell „Detection Transformer (DETR)“ [2] zur Fahrzeugklassifizierung im Straßenverkehr angewendet.
Erkenntnisse:
Im Hinblick auf die Prüfung von KI-Systemen besteht die zentrale Erkenntnis darin, dass eine standardisierte Prüfbeschreibung für KI-Systeme an spezifische KI-Bereiche (wie maschinelles Sehen oder natürliche Sprachverarbeitung) angepasst werden kann. Hier können zu den einzelnen Fachbereichen spezifische Prüfbeschreibungen inklusive Prüfschritten, Metriken und Festlegung erforderlicher Parameter formuliert werden. Der Mehrwert liegt aufgrund der vorgeschlagenen, einheitlichen Struktur in Nachvollziehbarkeit, einer homogen nutzbaren Kommunikationsgrundlage, optimierter Dokumentierbarkeit sowie Skalierbarkeit und Wiederholbarkeit von Prüfprozessen. Im Hinblick auf das KI-Gesetz fördert die Prüfung von Fähigkeiten gegen Gütekriterien die nachfolgenden Punkte:
- Transparenz und Nachvollziehbarkeit: Prüfung, unter welcher Güte Informationen wahrgenommen und verarbeitet werden, zur Schaffung von Transparenz in Entwicklung und Betrieb;
- Risikomanagement: Verdeutlichung von Schwächen und stabilen Betriebspunkten hilft, Risiken zu prognostizieren und zu vermindern;
- Menschliche Aufsicht und Interpretierbarkeit: Unterstützung der menschlichen Entscheidungsfindung durch Klarheit beim Betriebsverhalten;
- Verhinderung von Fehlanwendung: Das Verständnis über Leistbarkeit von KI-Systemen in spezifischen Szenarien hemmt Fehlanwendungen;
- Klarheit bei Kommunikation: Einheitliche Kommunikation über KI-Fähigkeiten zu Einsatzgrenzen von KI-Systemen.
Anwendbarkeit auf andere Projektkontexte: Die Testbeschreibung ist auf die breite Vielfalt heutiger KI-Systeme anwendbar, insbesondere auf solche, die einer Regulierung unterliegen sowie grundsätzlich auf Qualitätsgüte geprüft werden sollen. Grundsätzlich kann der vorgeschlagene Ansatz angepasst sowie auch erweitert werden im Hinblick auf nachfolgende Punkte:
- Adaptierbarkeit auf KI-Systeme: Das Schema eignet sich für die Prüfung verschiedener KI-Systeme, unabhängig von KI-Methoden- und Fachbereich;
- Nachweis regulatorischer Anforderungen: Besonders nützlich für KI-Betreiber sowie Marktüberwachungsbehörden, zur Nachvollziehbarkeit von Mängeln.
Die Anwendbarkeit könnte jedoch unter bestimmten Bedingungen eingeschränkt sein:
- Ressourcen: Umfassende Prüfungen können umfangreiche Rechenressourcen und Expertise erfordern;
- Testumfang: Die Wirksamkeit des Schemas hängt von der Transparenz des KI-Systems sowie der Spezifität der definierten Qualitätskriterien und Testszenarien ab;
- Systemkomplexität: Das Schema kann bei hochkomplexen oder neuartigen KI-Fähigkeiten, die über den aktuellen Stand der Technik hinausgehen, limitiert sein, was die Anwendbarkeit einer standardisierten Testbeschreibung erschwert.
Nutzen für meinen Arbeitgeber (Bundesnetzagentur): Im Kontext der KI-Regulierung kann eine standardisierte Testbeschreibung von KI-Systemen für die Nachvollziehbarkeit von Qualitätsmerkmalen vom Markt entzogener KI-Produkte förderhaft sein (Marktüberwachung).
Quellen:
[1] Beuth Verlag. (2023). Künstliche Intelligenz managen und verstehen:
Der Praxis-Wegweiser für Entscheidungsträger, Entwickler und Regulierer. Beuth Verlag.
[2] Hugging Face. (2024). Overview: The DETR model
huggingface.co/docs/transformers/model_doc/detr/ (Abgerufen am 16.10.2024)
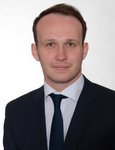
Taras Holoyad, Bundesnetzagentur
Nach dem Studium der Elektrotechnik an der Technischen Universität Braunschweig (M.Sc.) sammelte Taras Erfahrungen in der Automobilindustrie (Berechnung elektrischer Maschinen) und wechselte anschließend in die Normung von künstlichen Intelligenz. Derzeit liegt sein Schwerpunkt auf der Normung von künstlicher Intelligenz bei der Bundesnetzagentur auf nationaler Ebene (DIN/DKE) sowie weltweit (ETSI, ISO/IEC und ITU).
Seine einflussreichsten Projekte sind:
- Projektleiter von ISO/IEC 42102 „Taxonomy of AI system methods and capabilities“;
- Evaluierung der algorithmenbasierten Verkehrsklassifizierung am Beispiel der Stadt Wiesbaden;
- Mitautor des Schemas „AI=MC2“ und des dazugehörigen Buches
Schmid, T., et al. (2023). Künstliche Intelligenz managen und verstehen: Der Praxis-Wegweiser für Entscheidungsträger, Entwickler und Regulierer. Deutschland: DIN Media GmbH; - Mitautor des Online-KI-Glossars „AI-Glossary.org“.